Why do we need crop classification?
In agriculture, knowing what crops are planted and where can be an extremely valuable piece of information for traders, setting price points, and for ecological considerations such as nutrient inputs. Many countries around the world have little to no information on planted crops and in the United States, the locations of all crops are only released the following year by the U.S. Department of Agriculture. PlanetWatchers has developed a crop classification solution to identify a multitude of crop types anywhere in the United States earlier than ever before.
What is our technology?
David Braginsky (Algorithm Development Lead) explains, “Our machine learning, or more specifically, deep learning, models for crop classification provide pixel-based segmentation of an area of inspection for a given time period. To achieve better results we keep a set of pre-trained models and pick the most relevant for a customer’s needs. Moreover, it’s relatively easy to adjust existing models (e.g. by transfer learning) to new data, like new areas with their peculiarities, new crops, etc., making our approach both flexible and scalable. Another advantage is the potential to greatly improve the accuracy of a model with more ground-truth data from the region of interest using specific data. The averaged Dice coefficient (one of the ‘Intersection over Union’ estimators used to assess segmentation results) for our models is around 80%. Though it should be pointed out that it is feasible to achieve better results with more specific training data.”
PlanetWatchers uses multiple inputs of satellite imagery but is an expert in analyzing and processing Synthetic-Aperture Radar (SAR) imagery. Unlike optical imagery, which relies on the passive solar energy reflected off the Earth, SAR imagery employs an active sensor to emit microwave radiation. With wavelengths on the scale of millimeters and centimeters, SAR can collect data without solar illumination (at night) and is not affected by atmospheric conditions (clouds, fog, etc.), making it an ideal data source for constant and reliable monitoring of agricultural fields and planning data acquisitions well in advance.
How are satellites involved?
In the past, a lot of ground truthing was required to get a sense of the number of planted acres of various crops but in the last few years, the increasing number of available remote sensing sources have been utilized to attempt to solve this complex problem. As more and more satellites and smaller ‘CubeSats’ are launched and an increasing number of firms are battling to take advantage of this “New Space” revolution, we thought it would be a good idea to describe how PlanetWatchers has created crop classification algorithms and automated processes to classify crops as early as one month after emergence with an overall accuracy of 99% (Intersection over Union accuracy ~80%).
What is coming down the pipeline?
Working with many of the SAR sensors, PlanetWatchers collects massive amounts of data by automated integration into our cloud-based processing pipeline. A variety of databases and workflows have been incorporated into PlanetWatchers’ pipeline including MongoDB and StackStorm. These allow for the rapid ingestion of SAR imagery, which then moves to automated analysis in our Neural network frameworks.
How is PlanetWatchers scaling to more crops and geographies?
PlanetWatchers has trained and tested its crop classification algorithms in the United States and is soon expanding to other territories on multiple continents for additional crops of interest (over one dozen at the moment). We are not focused only on the large corn and soy industrial farms in the Midwest but also the one-acre farms all over the world in places like India and Africa providing food for a large percentage of the global population and characterized by poor timely data on crop type and acreage. Since we are always improving our algorithms, we have and will continue to be able to quickly scale this solution to any crop in any location under any environmental condition.
“The fact that our crop classification algorithms are built using SAR imagery means that as more and more SAR satellites become available in the near future, radiometry, spatial, and temporal resolution and the precision of our maps will only improve,” says Amihai Granot (Remote Sensing Lead at PlanetWatchers).
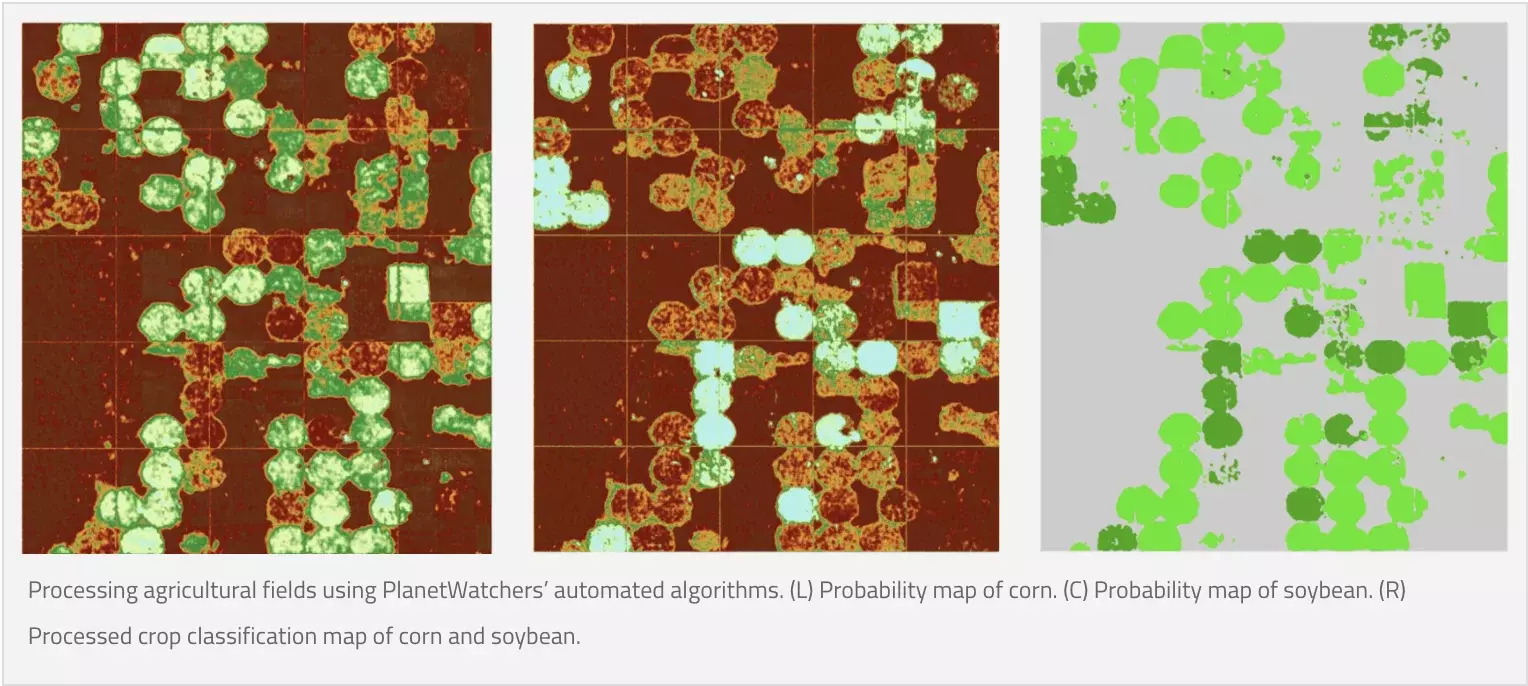
Processing agricultural fields using PlanetWatchers’ automated algorithms.
Focusing exclusively on crop insurance in North America, PlanetWatchers tells the story of every field saving our customers time and money by enhancing policy and claims validation.